In recent years, the world of machine learning (ML) has witnessed remarkable advancements, largely fueled by the availability of vast amounts of data. Data is the lifeblood of machine learning, driving everything from natural language processing (NLP) to computer vision and autonomous systems. However, there is one major challenge: data scarcity. For machine learning models to be accurate and effective, they require large volumes of high-quality, diverse, and well-labeled data—resources that can often be difficult, expensive, or time-consuming to gather.
This is where synthetic data enters the picture as a game-changer. Synthetic data, which is artificially generated rather than collected from real-world observations, has emerged as a promising solution to the data scarcity problem. With its ability to mimic real-world data without privacy concerns or data collection bottlenecks, synthetic data is poised to transform the future of machine learning.
In this article, we will explore what synthetic data is, how it works, the advantages it offers, and the potential challenges that come with its use. We’ll also delve into the question: Could synthetic data be the future of machine learning?
What is Synthetic Data?
Synthetic data refers to data that is artificially generated rather than obtained through direct measurement or real-world observations. It is typically created using algorithms, models, or simulations designed to replicate real-world phenomena. While synthetic data is not “real” in the traditional sense, it is designed to closely mirror the statistical properties and patterns of real-world data.
There are various methods for generating synthetic data, including:
- Generative Models: Machine learning models such as Generative Adversarial Networks (GANs) and Variational Autoencoders (VAEs) can generate synthetic data that looks and behaves like real-world data.
- Simulations: For some applications, synthetic data can be generated through complex simulations (e.g., autonomous vehicle testing or medical scenarios).
- Data Augmentation: Synthetic data can also be created through techniques like flipping, rotating, and cropping images, which is common in computer vision applications to create variations of real data.
The goal of synthetic data is to produce data that retains the essential patterns and structures needed for machine learning while avoiding some of the limitations and challenges associated with real-world data.
Why is Synthetic Data Important for Machine Learning?
The importance of synthetic data in machine learning can be summed up in several key ways:
1. Overcoming Data Scarcity
Real-world data is often expensive, hard to come by, or difficult to label. For example, obtaining labeled datasets for medical imaging, autonomous driving, or rare diseases can be particularly challenging. Synthetic data helps solve this problem by allowing organizations to generate data on demand, at scale, and without the constraints of real-world data acquisition.
2. Enhancing Data Privacy and Security
Synthetic data can be generated without any personally identifiable information (PII), making it particularly attractive for industries that deal with sensitive data, such as healthcare, finance, and telecommunications. For example, in the case of healthcare, generating synthetic patient data allows researchers and developers to create robust models without compromising patient privacy, thus addressing concerns related to data privacy regulations like GDPR or HIPAA.
3. Enabling More Robust Training
Machine learning models, particularly deep learning models, require large, diverse datasets to generalize well. However, real-world datasets are often limited in diversity. Synthetic data can be used to create variations that help improve model robustness. For instance, synthetic data can be used to augment datasets for image recognition by generating altered versions of existing images (e.g., rotating, scaling, or changing lighting), or it can be used to simulate rare events in a dataset to improve anomaly detection.
4. Cost-Effective
Data collection and labeling can be resource-intensive, especially for specialized fields like medical research or autonomous vehicle development. Synthetic data can be generated at a fraction of the cost, enabling organizations to scale their ML efforts more effectively. By supplementing or even replacing real-world data in some cases, synthetic data can save both time and money in the long run.
How Does Synthetic Data Work in Machine Learning?
Synthetic data works by simulating the characteristics of real-world data through algorithms or models. Depending on the type of synthetic data being generated, the methods can vary, but the general approach involves using statistical models, machine learning techniques, or simulations to create data points that resemble the original data set.
1. Using Generative Models (e.g., GANs)
Generative Adversarial Networks (GANs) are one of the most popular techniques for generating synthetic data. A GAN consists of two neural networks: a generator and a discriminator. The generator creates synthetic data, while the discriminator tries to distinguish between real and synthetic data. Through a process of back-and-forth training, the generator improves its ability to create realistic data that passes as “real” to the discriminator.
For example, in computer vision, a GAN might generate synthetic images of faces, animals, or even entire scenes. As the GAN improves over time, the synthetic images become nearly indistinguishable from real ones.
2. Data Simulation
For certain use cases, such as autonomous driving or industrial applications, synthetic data can be generated using simulations. In autonomous driving, companies use virtual environments like CARLA or Unreal Engine to create virtual driving scenarios with a variety of road conditions, obstacles, and weather patterns. These simulations allow autonomous vehicle algorithms to be trained without the need for real-world driving miles, which would be impractical and dangerous.
3. Data Augmentation
In some cases, synthetic data can be generated by augmenting existing datasets. This technique is common in computer vision, where existing images are transformed (flipped, rotated, cropped, or altered in color) to create variations. Data augmentation helps ensure that the model is trained on a wide variety of scenarios, improving its ability to generalize and perform well in real-world applications.
4. Synthetic Data for Privacy
For privacy-sensitive applications, such as healthcare, synthetic data is often generated by modeling the statistical properties of real datasets without using any actual personal data. This can involve creating entirely new datasets that maintain the same trends, distributions, and correlations as the original data while eliminating the risk of exposing sensitive information.
Advantages of Using Synthetic Data
1. Scalability
Synthetic data can be generated in large quantities on-demand, allowing organizations to scale their machine learning projects without the limitations imposed by the availability of real-world data.
2. Data Diversity
Synthetic data can be manipulated to include variations that might be underrepresented in real-world data. For instance, rare events, edge cases, or minority class samples can be generated to ensure that machine learning models are exposed to a broader range of scenarios, improving their robustness and reducing biases.
3. Faster Time to Market
By generating synthetic data on-demand, organizations can accelerate the development of their machine learning models. Data collection and labeling can be time-consuming processes, but with synthetic data, the training phase can be completed more quickly, leading to faster innovation and time to market.
4. Reduced Privacy Concerns
Synthetic data removes the privacy concerns associated with using real-world sensitive data. In sectors like finance, healthcare, or law enforcement, where strict regulations on data privacy exist, synthetic data allows organizations to innovate without risking compliance violations.
Challenges and Considerations
While synthetic data holds enormous potential, there are several challenges that need to be addressed before it can fully revolutionize machine learning:
1. Quality and Realism
One of the biggest challenges with synthetic data is ensuring that it accurately reflects real-world data. Poorly generated synthetic data that doesn’t capture the complexities of the real world can lead to models that perform poorly in production environments. Generative models like GANs are improving, but ensuring that synthetic data is both high quality and realistic remains a work in progress.
2. Bias in Synthetic Data
If the models used to generate synthetic data are based on biased or incomplete real-world data, the synthetic data will inherit those biases. This could lead to biased models that produce unfair or inaccurate results. It’s crucial to ensure that synthetic data is not only realistic but also diverse and unbiased.
3. Validation and Trust
Organizations must ensure that synthetic data is validated before it is used in machine learning applications. Unlike real-world data, which has been observed and collected over time, synthetic data needs to be thoroughly tested to ensure that it genuinely represents the diversity and complexity of real-world scenarios.
4. Data Availability for Specific Domains
In highly specialized fields, such as rare medical conditions or certain scientific research, generating sufficiently representative synthetic data can be particularly difficult. While synthetic data is great for certain use cases, it may not always be a viable substitute for real-world data in highly specialized or niche applications.
Why is machine learning the future?
Machine learning (ML) is no longer a buzzword—it is fundamentally reshaping industries, revolutionizing business practices, and enhancing everyday life. From autonomous vehicles to personalized healthcare, machine learning is playing a pivotal role in advancing technology and unlocking new possibilities. As we move into an increasingly digital world, the question arises:
Data Is Exploding, and Machine Learning Can Handle It
Machine learning thrives on data. Unlike traditional programming, where rules must be manually written to process inputs, ML algorithms can automatically learn patterns and relationships from large datasets, improving their accuracy over time. The ability of machine learning systems to process, analyze, and learn from vast quantities of data makes it indispensable in tackling the challenges posed by data overload.
Automation and Efficiency at Scale
Machine learning is a powerful driver of automation. It can help automate repetitive tasks, streamline processes, and reduce the need for manual intervention. By automating tasks that traditionally require human expertise, organizations can save both time and resources, allowing employees to focus on higher-level tasks that require creativity, judgment, and emotional intelligence.
For example, in customer service, ML-powered chatbots and virtual assistants are capable of handling a large volume of routine queries, freeing up human agents to handle more complex issues. In manufacturing, ML algorithms are used to optimize production lines, predict maintenance needs, and improve product quality.
Personalization and Customer Experience
Consumers today expect personalized experiences, whether they’re shopping online, using social media, or interacting with a service. Machine learning plays a crucial role in meeting these expectations. By analyzing data about consumer behavior, preferences, and interactions, machine learning algorithms can offer personalized recommendations, advertisements, and content tailored to individual users.
Some popular examples of machine learning-driven personalization include:
Social Media: Facebook, Instagram, and Twitter use machine learning to curate personalized content feeds and ads that align with users’ interests and engagement patterns.
E-commerce: Platforms like Amazon and eBay use ML to recommend products based on past browsing behavior, purchase history, and search queries.
Streaming services: Netflix, Spotify, and YouTube use machine learning to suggest movies, shows, or music based on user activity and ratings.
Machine Learning scope
Machine learning is a fast-evolving field with high demand for skilled individuals. By undergoing an artificial intelligence course or machine learning training, individuals can position themselves for lucrative prospects in different industries like technology, healthcare, finance, etc
Career Prospects:
Machine learning is a fast-evolving field with high demand for skilled individuals. By undergoing an artificial intelligence course or machine learning training, individuals can position themselves for lucrative prospects in different industries like technology, healthcare, finance, etc.
Stay Competitive:
In today’s digital era, businesses are using machine learning to acquire insights from data, automate processes, and improve decision making. By investing in ML courses, businesses can stay competitive by leveraging the power of data-driven solutions.
Innovation and Efficiency:
Machine learning allows businesses to innovate by developing new products, services, and solutions. By training employees in ML strategies, businesses can drive innovation and enhance operational efficiency via automation and optimization.
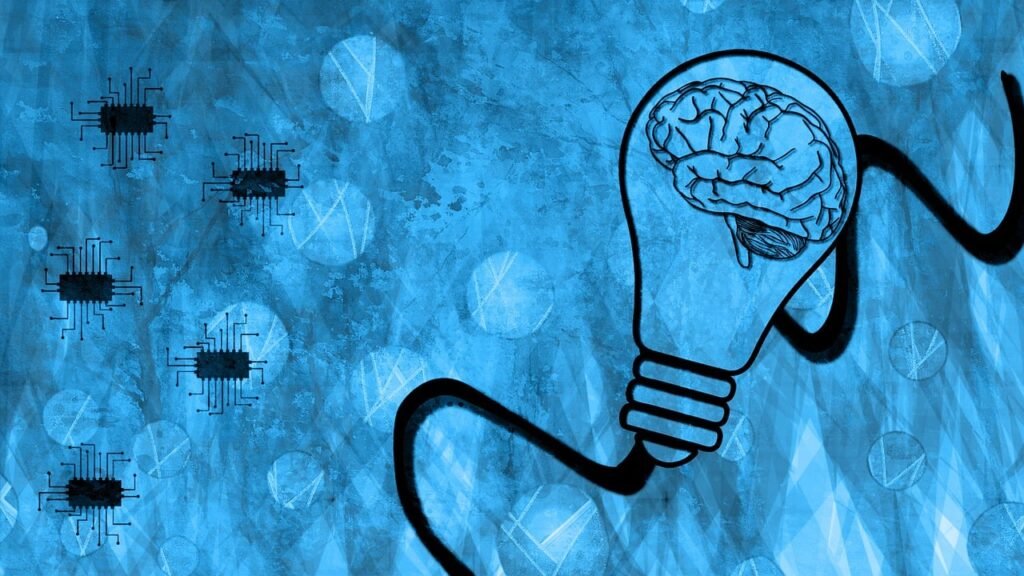
Data Utilization:
Machine learning courses empower individuals with the skills necessary to determine valuable insights from large datasets. This allows organizations to make data-driven decisions, determine patterns, trends, and anomalies, and acquire a deeper understanding of their customers, markets, and operations.
Problem Solving:
Machine learning provides strong tools and algorithms for addressing a wide range of complicated issues, such as predictive analytics, picture identification, and natural language processing. Individuals who participate in ML training can learn the abilities required to effectively address these difficulties.
So, I’m still questioning why I should go for machine learning training. Well, the answer is that the journey isn’t just about reaching a destination; it’s about embarking on a journey that promises to redefine what’s possible.